New benchmark for HQ transcriptions
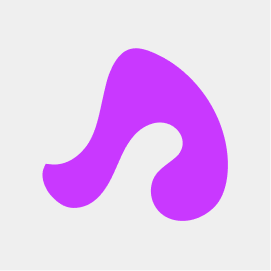

Advancements in automatic speech recognition (ASR) – aka speech-to-text – have led to huge strides in accuracy for automatic lyric transcription (ALT). In developing our ALT model, AudioShake’s Research team needs to regularly test how accurately our model can transcribe dialogue or vocals against industry benchmarks. The resulting performance metrics ultimately help evaluate the strength of ALT models.
However, when testing our model, our team found that public ALT benchmarks have focused exclusively on word content while ignoring the finer nuances of written lyrics including punctuation, line breaks, letter case, and non-word vocal sounds. These elements – which are covered by the guidelines of music industry leaders for lyrics including Apple, LyricFind, and Musixmatch – are important for high-quality lyric transcripts as they make lyrics more readable and help convey rhythm, emotional emphasis, rhyme, etc.
To address these issues, our team has introduced Jam-ALT. This new lyrics transcription benchmark, based on the JamendoLyrics dataset, implements our newly compiled annotation guide, which unifies the aforementioned industry guidelines. Our team also revised the dataset to account for spelling errors and incorrect or missing words. Besides the revised data, Jam-ALT also defines new automatic metrics that measure the accuracy of a transcription system in predicting punctuation, line/section breaks, and letter case, in addition to word error rate (WER).
The benchmark, presented at ISMIR 2023, enables more precise and reliable assessments of transcription systems and we hope that this helps advance lyrics transcription and improve the experience of generating lyrics for uses like live captioning or karaoke.
After developing Jam-ALT, our team ran various transcription systems against this new benchmark for evaluation. Notably, the results of Whisper v2 and v3 vary widely (possibly in part due to Whisper’s erratic behavior on music), but neither of them consistently outperforms the other. LyricWhiz (a system that uses ChatGPT to post-process Whisper’s output) fares much better in terms of word error rate, but not most of the other metrics. Finally, our in-house system consistently outperforms Whisper by a large margin (except for French).
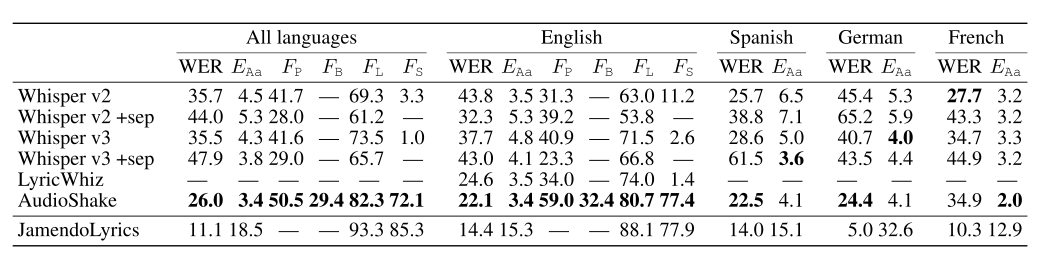
Benchmark results. WER is case-insensitive word error rate, EAa is case error rate, the rest are F-measures (P: punctuation, B: parentheses, L: line breaks, S: section breaks). “+sep” indicates vocal separation using HTDemucs. Whisper results are averages over 5 runs with different random seeds, LyricWhiz over 2 runs; our system (AudioShake) is deterministic, hence the results are from a single run. The last row shows metrics computed between the original JamendoLyrics dataset and our revision.
📄 Read the full paper on arXiv
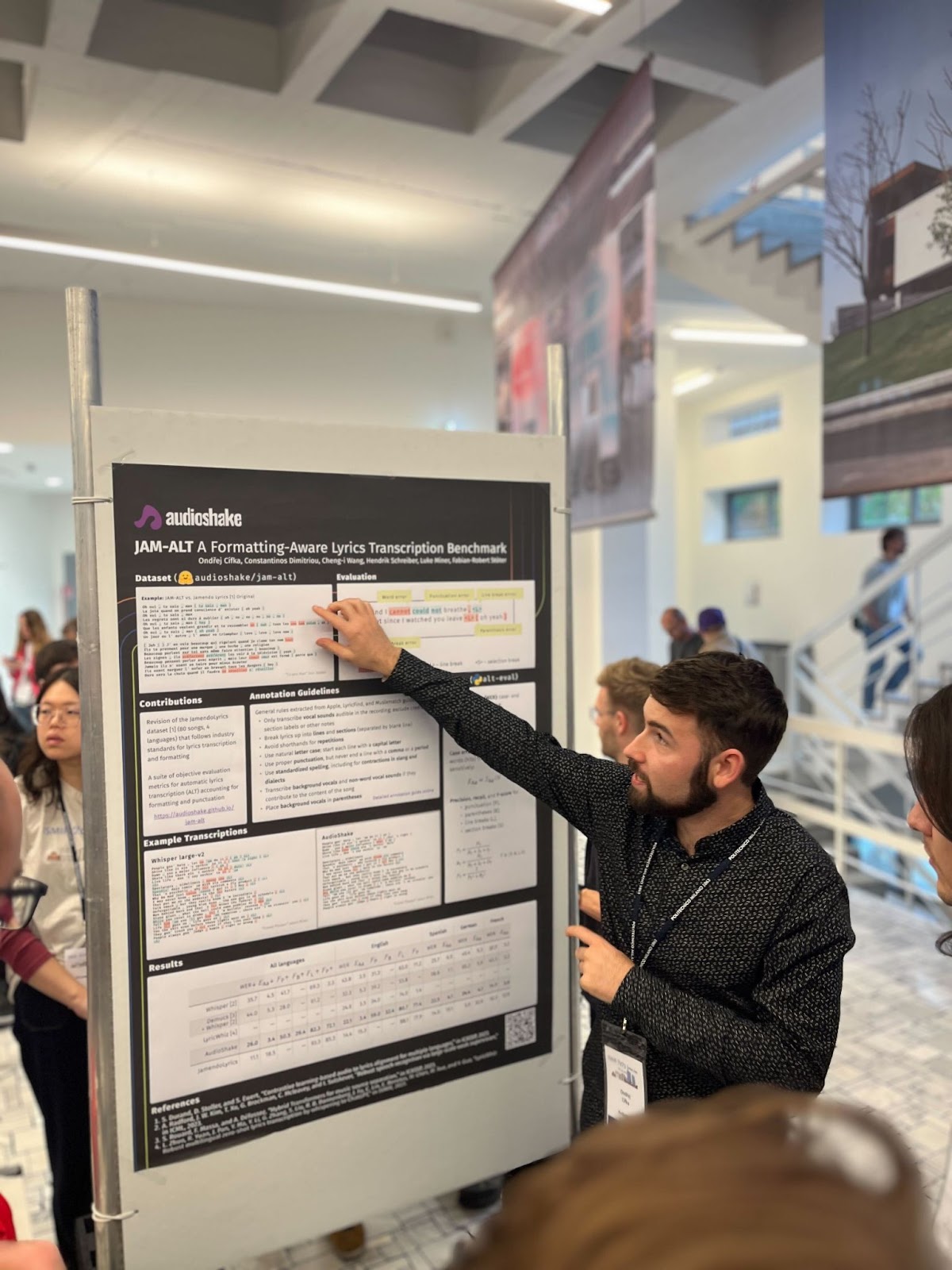
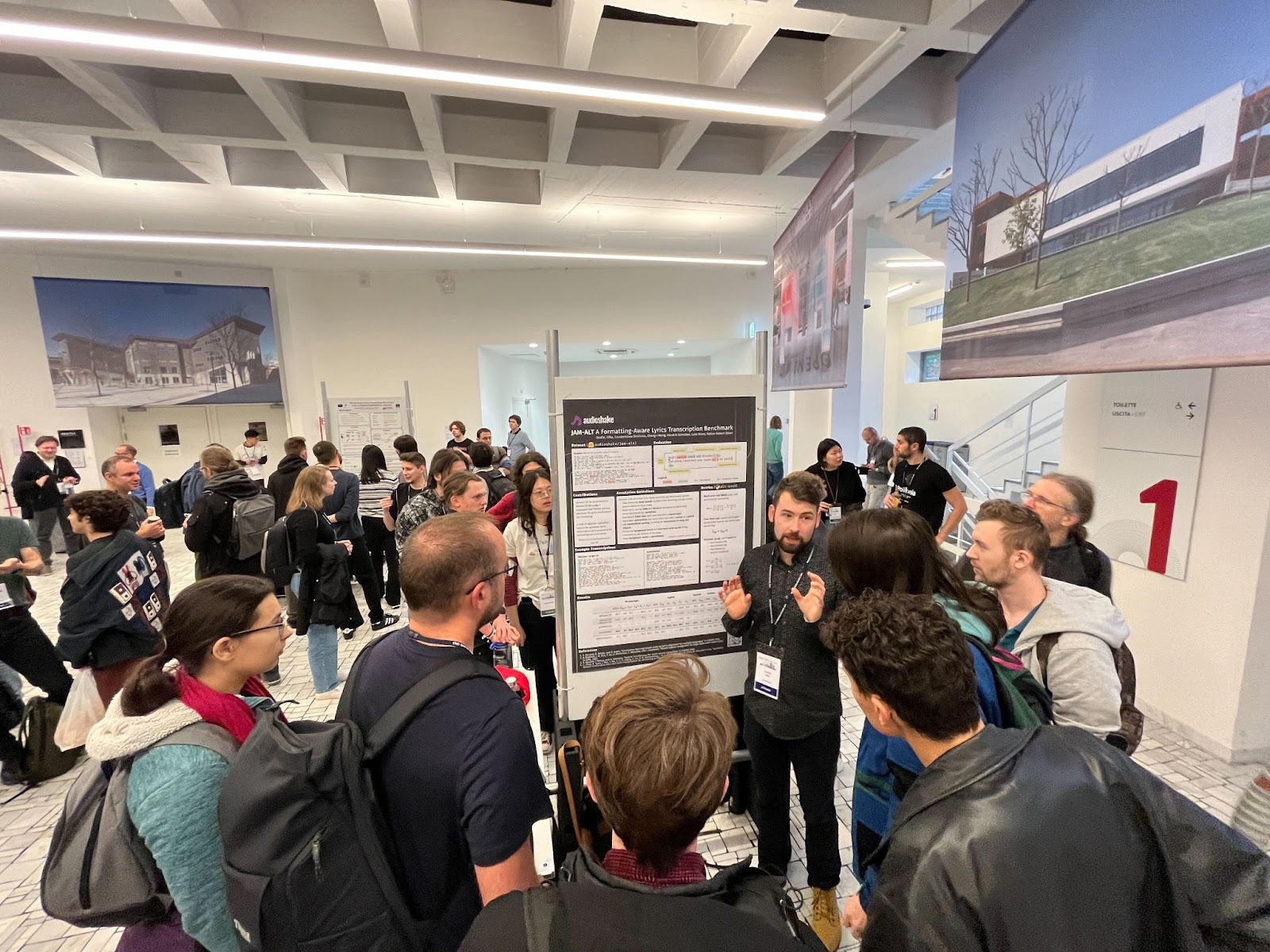